PhD Research
My PhD research was in the field of synthetic biology, with Timothy Lu at MIT.
I invented a method to generate and screen combinatorial genetic perturbations in O(1) time. Here’s what that means and why it’s significant.
Background
First, the basics. Genes are the foundation of our biology. They code for proteins that have functions in our cells and bodies, and these proteins interact with each other in dense networks. We understand only a small fraction of all the functions these genes likely have, and especially little about how they relate to disease. For example, the mechanisms of diabetes and heart disease are still frustratingly poorly understood.
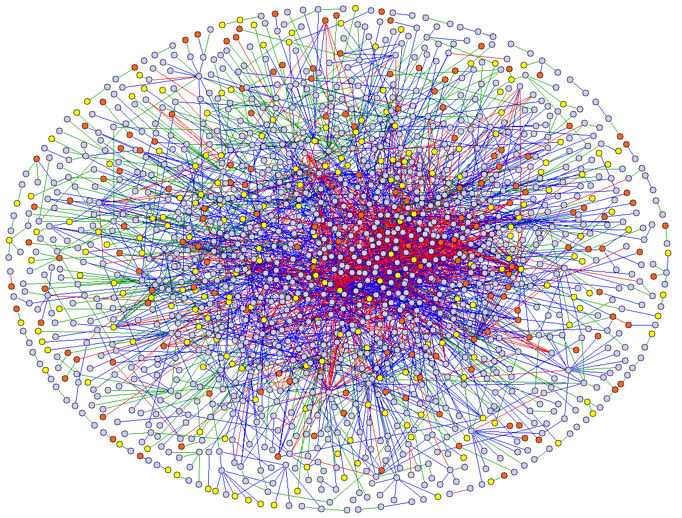
You can study the function of genes by artificially turning gene expression way up or way down (like a volume meter) in cells. For example, if you have a mysterious gene that you turn up, and suddenly the cells show signs of cancer, then there’s a decent chance the gene has something to do with cancer.
A powerful way to study biology is to run these studies across every single gene that you have. Humans have about 20,000 genes. It’d be really cool to crank each of these up, individually, and see what the effects are. For example, you might find that 300 of your 20,000 genes lead to a diabetes-like state – this is now a great starting point to dig deeper. This is called genome-wide screening and has already been heavily explored.
The more difficult and interesting question is – how do combinations of genes cause effects? Learning about single genes is useful, but remember that genes belong to networks, and networks drive biology. If you can study how combinations of genes cause disease, then you get a much clearer picture. For example, out of your 300 genes that led to diabetes above, if you found that 10 pairs, when cranked up together, led to a super-severe diabetic state, then this would be an even better place to start. You’d also find surprising combinations of genes that interact with each other that you couldn’t have predicted a priori.
Now here lies the problem – creating and studying these combinations is incredibly labor intensive. Using traditional techniques, you would need to create and study every single one of those combinations separately. For example, using the most brute-force method, if you want to create pairwise combinations of every gene with each other, that would require 20,000 x 20,000 = 400 million separate experiment setups. This would require equipment on the size of a football field, let alone the prohibitive costs of reagents and labor. (In computer science terms, this is O(n2) time).
And higher-order combinations above pairwise – forget about it. A 3-wise combination would require 8 trillion experiments, and a 4-wise combination would require 160 quadrillion experiments. Thus, if you wanted to run an m-wise combination experiment, this would require O(nm) time.
The Solution
And so, finally, my research (as with much of science, it takes a long time to explain why the research actually matters).
In short, I invented a method that allows you to generate large combinations of genes and study their effects in an incredibly short period of time. Specifically, creating the initial library of genes take O(n) time, and scaling to each combination afterward takes O(1) time. This is a big improvement over O(nm) time.
Functionally, this means:
- the most laborious step is the first one: creating the gene expression units. For every gene you want to study (and crank up or down) you need to create it manually. Say we want to study 2,000 genes – this will require creation of 2,000 separate gene units. (O(n) step)
- to create a pairwise combination, we need to do only ONE reaction in ONE tube. This creates all 4,000,000 pairwise combinations in one combined population.
- to experiment with this population, we just need to inject each combination into a cell. This is usually done via transformation or transfection.
- to create a 3-wise combination, we again need to do only ONE reaction in ONE tube. This creates all 8,000,000,000 3-wise combinations in one tube
This means you could create 1 million 3-wise combination variants in a single afternoon in one reaction, instead of requiring a million separate reactions. Afterward, you can study the entire population in a single tube, instead of needing a million separate reaction wells.
Technical Details
The technical details of this are:
- each genetic construct (eg an inducible overexpression or knockdown unit) is created in a plasmid and labeled with a genetic barcode
- the barcode and the construct have restriction sites in specific places to allow splicing in of other barcoded constructs
- after a digestion and ligation reaction, the barcodes are now in tandem, as are the genetic constructs. This graphic will clarify things:
- this reaction can be iterated to create 3-wise, 4-wise, 5-wise, etc. combination libraries
- the barcodes in sequence can be read via Illumina HiSeq, allowing one-pot reactions and assays
- these plasmid libraries are transformed into cellular populations
- by comparing an experimental induced population with a control population, you detect changes in the frequency of barcode combinations across the population. This can suggest synergies between genes specific to your phenotype
- for example, if you were interested in proliferation as a phenotype, genetic combinations that became over-represented would suggest a boost to proliferation, whereas combinations that disappeared may represent toxic combinations
- this is a generalized technique applicable to cells of your choice, from bacterial to human
Using this method, you can find unexpected synergies between genes, as well as study interactions at the network level.
Limitations of the Technique
The above description is a simplification, and as with all techniques there are limitations that cap how many unique combinations you can really study:
- the transformation/transfection efficiency limits how many unique library members you can get into a population. In bacteria, you might get 106 transformants per reaction, which means even if you have 108 unique library members in plasmids, most won’t make it into the population. You can of course run more transformations, then pool the population to form your experimental population
- the Illumina HiSeq currently caps at around 5 billion reads, and to detect differences in your population, you’ll want to be able to sample each unique combination multiple times
So, for now, it’s still difficult to assay trillions of combinations, but you can get into the hundreds of thousands and millions reasonably.
Where’s the Research Now?
I published my thesis work in PNAS in 2013, where I looked for combinations of genes that would overcome antibiotic resistance. We also patented the technique, now called “massively parallel combinatorial genetics” or “CombiGEM – Combinatorial Genetics en Masse.”
CombiGEM has since been applied to chemotherapy resistance and to CRISPR for discovery of effective drug combinations. It’s also the core technology behind Engine Biosciences.
There are more cool applications of CombiGEM that would be cool to do:
- combine targets of FDA-approved drugs, to discover unexpected drug synergies to treat disease
- discover combinations of transcription factors that lead to stem cell differentiation to your endpoint of choice. This is usually done manually in a labor-intensive, 1-by-1 gene elimination method
- combine genes implicated in GWAS studies to find interactions that lead to disease. This helps address the issue that GWAS genes don’t explain much of the heritability of disease
Hacking Medicine
Healthcare’s a tough industry to innovate in, requiring the combined expertise of technologists, health practitioners, and business operators. But these people rarely have chances to intersect. This means builders don’t know what health problems to solve, while practitioners know what problems exist but don’t know how to create a sustainable solution.
So in 2011, I co-founded Hacking Medicine with Elliot Cohen and Zen Chu, creating one of the first health hackathons. The idea was simple – bring engineers, doctors, and businesspeople together over one weekend to hack on health problems. Few projects actually became real companies, but the important result was creating a community around healthcare innovation and building working relationships that last for years.
Since then, Hacking Medicine’s grown to become an incredible community of healthcare innovators worldwide. The organization partners to put on dozens of health hackathons worldwide, as well as putting on the flagship annual Grand Hack at MIT.
Why I Left Medicine for Business
I get a lot of questions about why I went through all the trouble of getting into an MD-PhD program, just to drop out before getting either degree. My parents were certainly alarmed!
I’ll share my own journey and lessons; this is targeted particularly toward students and young adults.
In short, over my young adult life, I tried out a lot of different things and reflected constantly about what I liked and disliked about them. This let me learn what I really wanted out of life—on my deathbed at 90 years old, what would make me look back and think, “I really could not have spent that time any better, and I have no regrets”?
It turned out medicine and science were both worse options for me than starting businesses. So I left the entire medical/scientist track and never turned back.
My Life Preferences
Let’s cheat and jump to the answer first: what do I personally want out of life? It took years and lots of projects to learn these:
- I want to have a scalable, nonlinear impact. My impact should grow exponentially with time and effort; I don’t want a linear impact, because this feels limiting. A related concept is leverage – I want to build big levers.
- I have to work on things that I feel uniquely suited to do. I like building products that wouldn’t exist if I didn’t build them; I hate building me-too products that are just carbon copies. Building unique things makes me feel like my existence was worthwhile—if I’m doing what any other person can do, did it really matter to the world if I existed?
- I need a lot of personal agency. I don’t like people telling me what to do. I bristle under authority and I don’t like having bosses.
- I like solving practical problems and making my and other people’s lives better. I don’t like working on things that are just theoretically interesting but don’t have a practical output (like theoretical math).
- I want to have an impact in a short time frame. I know my time on Earth is short, and I can die any time. I don’t want to wait 20 years to make a mark.
- I like learning new things. I don’t like doing a repetitive thing over and over again. Where some people like being specialists, I like being a generalist.
Some of these attributes were with me for a long time—I’ve had a rebellious, contrarian attitude since I was a kid, and I’ve mulled constantly on the point of my life since I was 5 years old. Other attributes I only discovered after time and experience—I only learned that I like building new things when I tried copying another company’s product and had an absolutely miserable time.
Evaluating Careers
Let’s now look at medicine and science through these lenses.
Medicine (specifically, practicing as a physician):
- Limited impact: your impact is linear—you see x patients a day for y years, and you have a linear impact. If you stop seeing patients, you stop growing your impact.
- Replaceability: As a, say, cardiologist, I could be totally replaced by any other cardiologist and the world would barely tell any difference. I didn’t think I could become a uniquely good cardiologist.
- Lack of agency: As a pure practitioner, there’s little room for innovation. In fact, deviating from guidelines is called malpractice. You’re also usually at the mercy of your employer and reimbursement system. The major agency you have is around discretionary clinical decisions.
- Repetitiveness: For the most part, practicing medicine is following a series of flowcharts to figure out what’s wrong with the person and how to fix it. It felt very mechanical and repetitive; in medical school, I realized being a doctor is like being a human mechanic. It’s very valuable—the world needs mechanics of all kinds—but it’s not my preferred working style.
Science: I actually liked science quite a lot. As a synthetic biologist/bioengineer, inventing new techniques and breaking new ground ticked a lot of boxes—scalable impact, unique work, personal agency, learning new things. The major issue was time frame—it can take 30 years to get from a laboratory discovery to real-world impact. And because it takes so long, it takes a ton of resources, which means you have to raise money and get your ownership diluted (decreasing agency and giving you bosses). I respect the hell out of people who can push the ball this long; I’m impatient and want results sooner.
Business: starting new businesses and building new products ticks all the boxes for me:
- Impact: I can build products that do far more than I could do with my brute force. A lot of this has to do with software and Internet technology—write software once, and it can be used by a billion people. Furthermore, I can build organizations of people who can achieve far more than I can do myself.
- Uniqueness: I choose to build products that I feel wouldn’t exist if I didn’t choose to build them.
- PrepScholar was the first test prep company that really did adaptive education right in that industry (in my biased opinion); we were also first to publish thousands of free, high-quality guides on high school and college success.
- Shortform summarizes information at a quality that I’ve seen no other company do, and our vision to cover every major idea in every form of media is something I haven’t seen exist yet.
- In fact, I often start companies by solving my own problems in ways that aren’t solved by what’s on the market; if the solution already existed, it would cease to be a problem for me, and I wouldn’t think to start a company around it.
- (I recognize there’s hubris in this, and it’s wholly possible that the products I build aren’t actually all that unique, or if they are that someone would have created it soon enough even if I didn’t exist. Even without Elon Musk, the world would have gotten to electric cars and landable rockets at some point.)
- Agency: as owner of my companies, I have a lot of freedom around what I choose to do and how I do it. This is especially true since I currently create companies with my own funds and don’t have outside investors. My real “boss” is my customers—I need to build things that solve their problems, and they can “fire” me if I don’t do this well enough.
- Short time frame: I can spin up a new product and company within months. And everyday that we have customers, we are actively solving people’s problems and making an impact.
- Learning new things: Being a company founder requires you to learn a bit of everything—product, engineering, marketing, operations, management, legal. To grow your company or enter new industries, you often have to learn things you’re totally new to. A week doesn’t pass where I don’t learn a new tool to add to my toolkit; every year I look back and think the Allen of the previous year was woefully underinformed.
Once again, it took me a while to get to these realizations, but once I learned them, it became impossible to do anything but what I wanted.
I should point out that these are my personal reflections based on my preferences. My best friends from med school don’t give a crap about having an exponential impact. And it doesn’t have to do with intelligence or ambition—they’re equally smart and want to be top of their fields—they just want different things out of life. That’s OK.
This also speaks to the importance of defining what YOU want out of life, not what your parents or friends say you should want, and not what I wrote above. It’s very easy to fool yourself into thinking you want what other people want—we’re social creatures, and we crave validation and belonging.
The Journey of Discovery
As I mentioned, discovering all these things took a long time – over a decade, from my time in high school to deep into my startup career.
For me, it was an organic path made up of two key activities: 1) trying orthogonal things that show you things you’ve never tried before, 2) introspecting on why I liked something and didn’t.
A sample of key experiences that taught me valuable lessons:
- Building new organizations (clubs, competitions) in college taught me how much I enjoyed going from zero to one and building teams
- Doing science research taught me the joy of uncovering things that the world hadn’t previously known
- Building my first business at the end of college taught me how much I enjoyed the personal freedom, business strategy, and the process of serving customers
- Going to medical school taught me how repetitive and unoriginal medicine could be, and how much it felt like being a mechanic of humans
- Doing a bioengineering research rotation taught me the joy of building things, beyond just uncovering the natural world through science
- Building a business taught me how much I enjoyed being a generalist and being competent at a lot of different things
- Building a replica of another business taught me how miserable purely copying other ideas made me feel
All my experiences taught me valuable things that helped me hone in on what I really enjoyed and why. It’s like playing the game Battleship—you try out one thing, it’s a hit or a miss, and you reflect on why and decide what to do next.
I didn’t approach this systematically, with a blueprint of what to do and when. It was much more organic. If I felt miserable, I thought about why that was and tried to avoid similar situations in the future. If an opportunity came along with some aspect that was totally new and a little scary, I usually took it on.
I certainly wish it didn’t take me this long to find my life answers. Had I known what I know now in high school, I could have saved a lot of time and achieved a lot more by now. I do think I could have started self-examination earlier and questioned what I wanted out of medicine earlier.
But, for the most part, it takes time and a lot of experimentation. Some people find it right away, some people take decades to find it, and many never find it (hence the mid-life crisis).
Self-discovery is also path-dependent. It’s very possible that if I started off being interested in business in high school, a string of startup failures could have led me to seek security and stability at a big company (or medicine) instead. I am who I am today because of a series of experiences in a particular order, mixed in with my personality. Experiencing this and discovering more about yourself is one of the joys of life.
Changing Tracks
My final piece of advice: if you know a change has to be made because you’re miserable, then take steps to change it. Don’t be trapped by your sunk costs.
Leaving my medical track was difficult. I’d thought I’d wanted to be a neurosurgeon since I was in middle school. I’d geared my entire college experience around getting into an MD-PhD program. I’d taken a fellowship from the US government that paid for my medical school tuition. When I was leaving, my school advisors told me it was a terrible idea. My parents thought business was too risky, and they wanted more stability for me (a common immigrant mentality). There was even guilt—”you have a spot in Harvard Medical School, something hundreds of thousands of people dream of, and now you’re throwing that away?” There were a lot of external forces against my leaving med school, and only a few internal ones for it.
But those internal reasons were strong. First, I knew I wasn’t as happy as I could be, and I felt it. I didn’t enjoy medical school lectures, and I didn’t relish what I was learning like my friends did (I especially thought anatomy dissections were a huge waste of time, while my best friends were thrilled each day). In my PhD years, I’d wake up the morning of a long day of experiments and stare at the ceiling in the cold Boston winter for 20 minutes before getting up. When I asked myself what my ideal life would be, it was as an entrepeneur who could build cool, world-changing products. The dissonance made it really clear that I was on the wrong track.
Second, the fear of regret outweighed the discomfort of making the change. For me, regret is the worst feeling to have in life. Regret is the feeling that, at the time, you knew you should have done something different, but you didn’t for some dumb reason, and now it’s too late. Regret eats at you for a lifetime.
When I was in graduate school, I knew that the longer I waited, the harder it’d be to leave. The younger I was, the more risks I could take, the more failures I could endure. All things considered, despite the opposition, I knew I had to make the change, and that made it easy.
I have plenty of colleagues from medical school who are practicing physicians today but are absolutely miserable. They know they don’t enjoy practicing medicine, but they feel it’s too late to change. A few key reasons for this:
- they’ve been on the medical track for so long that they’re scared to leave it. It means changing their identity, disappointing their parents, facing embarrassment, not being able to pay off their debt, not being able to support their young families.
- because they haven’t experimented with a lot of other things, they don’t know what else they’d do.
I understand most people have obligations and can’t just drop a career instantaneously. But, at the least, misery is a sign that you should find a better answer. Take the painful steps to find it—it’s worth it.